PaperView - Learn by Challenging Yourself Contrastive Learning with Hard Sample Generation
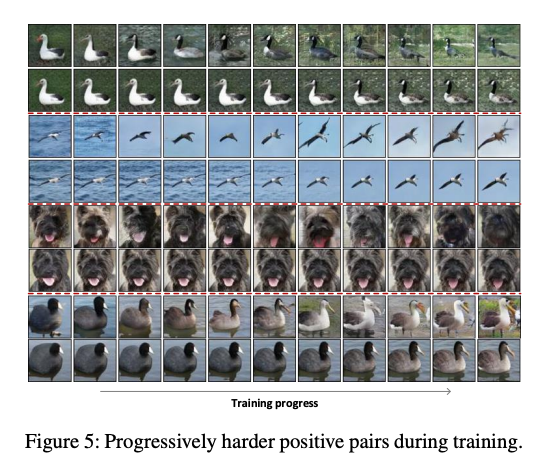
Please checkout my video explaining this interesting paper by clicking on the “Video” button above.
Abstract:
Contrastive learning (CL), a self-supervised learning approach, can effectively learn visual representations from unlabeled data. Given the CL training data, generative models can be trained to generate synthetic data to supplement the real data. Using both synthetic and real data for CL training has the potential to improve the quality of learned representations. However, synthetic data usually has lower quality than real data, and using synthetic data may not improve CL compared with using real data. To tackle this problem, we propose a data generation framework with two methods to improve CL training by joint sample generation and contrastive learning. The first approach generates hard samples for the main model. The generator is jointly learned with the main model to dynamically customize hard samples based on the training state of the main model. Besides, a pair of data generators are proposed to generate similar but distinct samples as positive pairs. In joint learning, the hardness of a positive pair is progressively increased by decreasing their similarity. Experimental results on multiple datasets show superior accuracy and data efficiency of the proposed data generation methods applied to CL. For example, about 4.0%, 3.5%, and 2.6% accuracy improvements for linear classification are observed on ImageNet-100, CIFAR-100, and CIFAR-10, respectively. Besides, up to 2× data efficiency for linear classification and up to 5× data efficiency for transfer learning are achieved.