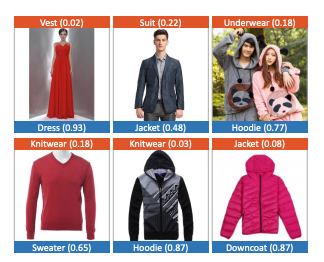
Please checkout my video explaining this interesting paper by clicking on the “Video” button above.
Abstract:
Despite the success of deep neural networks (DNNs) in image classification tasks, the human-level performance relies on massive training data with high-quality manual annotations, which are expensive and time-consuming to collect. There exist many inexpensive data sources on the web, but they tend to contain inaccurate labels. Training on noisy labeled datasets causes performance degradation because DNNs can easily overfit to the label noise. To overcome this problem, we propose a noise-tolerant training algorithm, where a meta-learning update is performed prior to conventional gradient update. The proposed meta-learning method simulates actual training by generating synthetic noisy labels, and train the model such that after one gradient update using each set of synthetic noisy labels, the model does not overfit to the specific noise. We conduct extensive experiments on the noisy CIFAR-10 dataset and the Clothing1M dataset. The results demonstrate the advantageous performance of the proposed method compared to state-of-the-art baselines.